Updated: Feb 28 2022
In this blog post we describe two case studies to illustrate improvements in speech-to-text or ASR recognition accuracy that can be expected from training of the underlying acoustic models. We trained our acoustic model to recognize Indian and Irish English better.
Case study setup
The Voicegain out-of-the-box Acoustic Model which is available as default on the Voicegain Platform had been trained to recognize mainly US English although our training data set did contain some British English audio. The training data did not contain Indian and Irish English, except for maybe accidental occurrences.
Both case studies were performed in an identical manner:
- Training data contained about 300 hours of transcribed speech audio.
- Training was done to get improved accuracy on the new type of data but at the same time to also retain the baseline accuracy. An alternative would have been to aim for maximum improvement on the new data at expense of accuracy of the baseline model.
- Training was stopped after significant improvement was achieved. It could have been continued to achieve further improvement, although that might have been marginal.
- Benchmarks presented here were done on data that was not included in the training set.
Case Study 1: Indian English
Here are the parameters of this study.
- We had 250 hours of audio containing male and female speakers, each speaker reading about 50 minutes worth of speech audio.
- We separated 6 speakers for the benchmark, selecting 3 male and 3 female samples. Samples were selected to contain both easy, medium, and difficult test cases.
Here are the results of the benchmark before and after training. For comparison. we also include results from Google Enhanced Speech-to-Text.
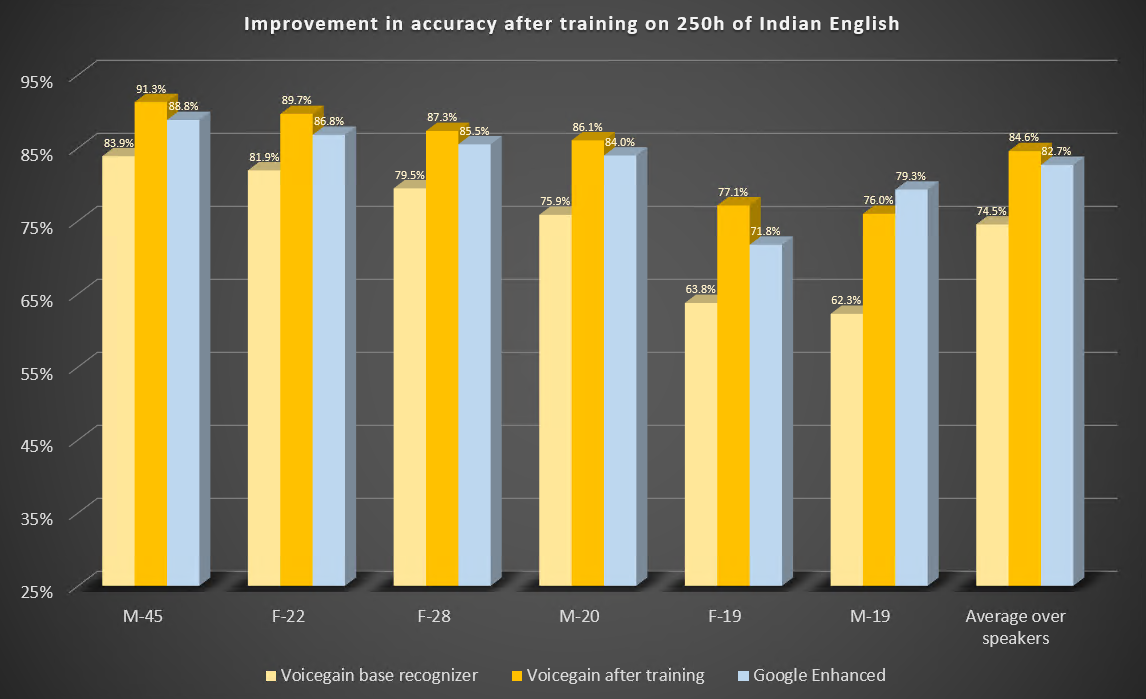
Some observations:
- All 6 test speakers show significant improvement over the original accuracy.
- After training the accuracy of 5 speakers is better than Google Enhanced Speech-to-Text. The one remaining speaker improved a lot (from 62% to 76%) but the accuracy was still not as good as Google. We examined the audio and it turns out that it was not recorded properly. The speaker was speaking very quietly and the microphone gain was set very high - this resulted in the audio containing a lot of strange artifacts, like e.g. tongue clicking. The speaker also ready the text in a very unnatural "mechanical" way. Kudos to Google for doing so well on such a bad recording.
- On average custom-trained Voicegain speech-to-text was better by about 2% on our Indian English benchmark compared to Google Enhanced recognizer.
Case Study 2: Irish English
Here are the parameters of this study.
- We collected about 350 hours of transcribed speech audio from one speaker from Northern Ireland.
- For the benchmark we retained some audio from that speaker that was not used for training plus we found audio from 5 other speakers with various types of Irish English accents.
Here are the results of the benchmark before and after training. We also include results from Google Enhanced Speech-to-Text.

Some observations:
- The speaker that was used for training is labeled here as 'Legge'. We see huge improvement after training from 76.2% to 88.5% which is significantly above Google Enhanced with 83.9%
- The other speaker with over 10% improvement is 'Lucas' which has a very similar accent to 'Legge'.
- We looked in detail at the audio of the speaker labeled 'Cairns' who had the least improvement and for whom Google was better than our custom trained recognizer. The audio has significantly lower quality that the other samples plus it contains noticeable echo. Its audio characteristics are quite different from that audio characteristics of the training data used.
- On average custom trained Voicegain speech-to-text was better by about 1% on our Irish English benchmark compared to Google Enhanced recognizer.
Further Observations
- The amount of data used in training at 250-350 hours was not large given that normally Acoustic Models for speech recognition are trained on 10s of thousands of hours of audio.
- The large improvement on 'Legge' speaker suggest that if the goal is to improve recognition on very specific type of speech or speaker the training set could be lower, maybe 50 to 100 hours, to achieve significant improvement.
- Bigger training set may be needed - 500 hours or more - in cases where the variability of speech and other audio characteristics is large.
UPDATE Feb 2022
We have published 2 additional studies showing the benefits of Acoustic Model training:
- Getting high Speech Recognition Accuracy on Alphanumeric Sequences: A Case Study with UK Zip Codes
- Model Training Delivers Huge Gains in Accuracy - Case Study: Indian Food Bot
Interested in Voicegain? Take us for a test drive!
1. Click here for instructions to access our live demo site.
2. If you are building a cool voice app and you are looking to test our APIs, click hereto sign up for a developer account and receive $50 in free credits
3. If you want to take Voicegain as your own AI Transcription Assistant to meetings, click here.